AI Updates
There is a lot of chatter about 2025 being the year of agentic frameworks. To me, this means a system in which a subset can allow AI models to take independent actions based on their environment, typically interacting with external APIs or interfaces.
The terminology around this concept is still evolving, and definitions may shift in the coming months—similar to the shifting discussions around Open Table Formats. Below are some key takeaways from recent discussions on agentic systems:
- Agents can take actions allowed by a given environment
- Agents can be potentially dangerous if given the ability to perform write actions
- Agent workflows can potentially be very expensive if not constrained (EG excessive token usage)
- Evaluating success on agent workflows is tricky.
- Chip Huyen recently wrote a new book on AI Engineering and created a standalone blog post about agents https://huyenchip.com//2025/01/07/agents.html
- Anthropic has explored agentic systems in their article: https://www.anthropic.com/research/building-effective-agents
When writing traditional software, it’s helpful to think in terms of determinism. In probability theory:
- P(1) represents an outcome that is guaranteed to occur (100%).
- P(0) represents an outcome that will never occur (0%).
Similarly, code is deterministic—given the same input, it will always produce the same output. Business rules drive execution, either directly through the code or abstracted into configuration files.
However, agents break this determinism by introducing an element of decision-making and uncertainty. Instead of executing predefined logic, agents operate within a framework that allows for flexible responses based on real-time data. This can be powerful, as it enables systems to handle edge cases without explicitly coding for them. But it also raises evaluation challenges—how do we determine if an agent is performing well when its behavior isn’t fully predictable?
Taking a data engineering perspective on this, I think usefulness of agents will be directly proportional to the quality of the data sources available for an agent.
- ChatGPT and similar LLMs have been useful primarily because they have likely ingested the entire Internet. Whether this has been done in full compliance with copyright laws is a separate issue.
- More structured domains, such as coding, have thrived due to the constraints imposed by programming languages, as these languages are inherently built upon specific grammatical rules.
When customers add data sources to their agentic frameworks I can only imagine a lot of data cleansing and structuring will have to be done to make these systems successful.
Other interesting links to learn about AI
re:Invent Recap
Every year, there are tons of announcements, and you can catch a recap of all the new services in this 126 slide PDF from AWS courtesy of community days.
https://assets.community.aws/a/reCap-Summary-2024.pdf
Data Highlights:
- Glue 5.0 – be sure to use this new latest version
Sagemaker Unified Studio
Another major launch during re:Invent was Sagemaker Unified Studio. Capabilities include:
- Amazon SageMaker Unified Studio (public preview): use all your data and tools for analytics and AI in a single development environment.
- Amazon SageMaker Lakehouse: unify data access across Amazon S3 data lakes, Amazon Redshift, and federated data sources.
- Data and AI Governance: discover, govern, and collaborate on data and AI securely with Amazon SageMaker Catalog, built on Amazon DataZone.
- Model Development: build, train, and deploy ML and foundation models, with fully managed infrastructure, tools, and workflows with Amazon SageMaker AI.
- Generative AI app development: Build and scale generative AI applications with Amazon Bedrock.
- SQL Analytics: Gain insights with the most price-performant SQL engine with Amazon Redshift.
- Data Processing: Analyze, prepare, and integrate data for analytics and AI using open-source frameworks on Amazon Athena, Amazon EMR, AWS Glue, and Amazon Managed Workflows for Apache Airflow (MWAA).
In the data field, there are two options, build vs buy. AWS traditionally follows the build approach, offering tools for developers to create their own platforms. However, operationalizing data platforms is complex, and competitors like Databricks and Snowflake offer faster onboarding workflows with vertically integrated components.
SageMaker Lakehouse shows potential as it attempts to bridge the gap between build and buy solutions. It offers robust management features within its UI, including CI/CD workflows similar to GitHub. However, given its early stage, I would approach this large platform with your primary use cases, and do a proof of concept first before approaching any production workloads.
However my main complaint is this service should never been called Sagemaker Unified Studio to begin with. Historically Sagemaker has been associated with the AI aspect of AWS, so it is easy to be confused that the new portfolio now includes data engineering. If I had to do it all over I would have called it
- AWS Data Unified Studio which has:
- Data Engineering Workflows
- Sagemaker AI Workflows
- Catalog Workflows
Open Table Format Wars
Industry has been talking about the Open Table Format (OTF) wars for a while, and really the problem has been more of a political problem rather than a technological one.
As a reminder, there are 3 major players in the space, Apache Hudi, Apache Iceberg, and Databricks Deltalake. In the past months, AWS, Databricks, and Snowflake have begun to coalesce around allowing catalogs based off Apache Iceberg to query and write to each other.
At its core, Apache Iceberg is a set of specifications. In this case, both the AWS Glue Data Catalog and Databricks have implemented the Apache Iceberg REST contracts, enabling them to query each other. AWS facilitates this by exposing an external endpoint that other vendors can access.
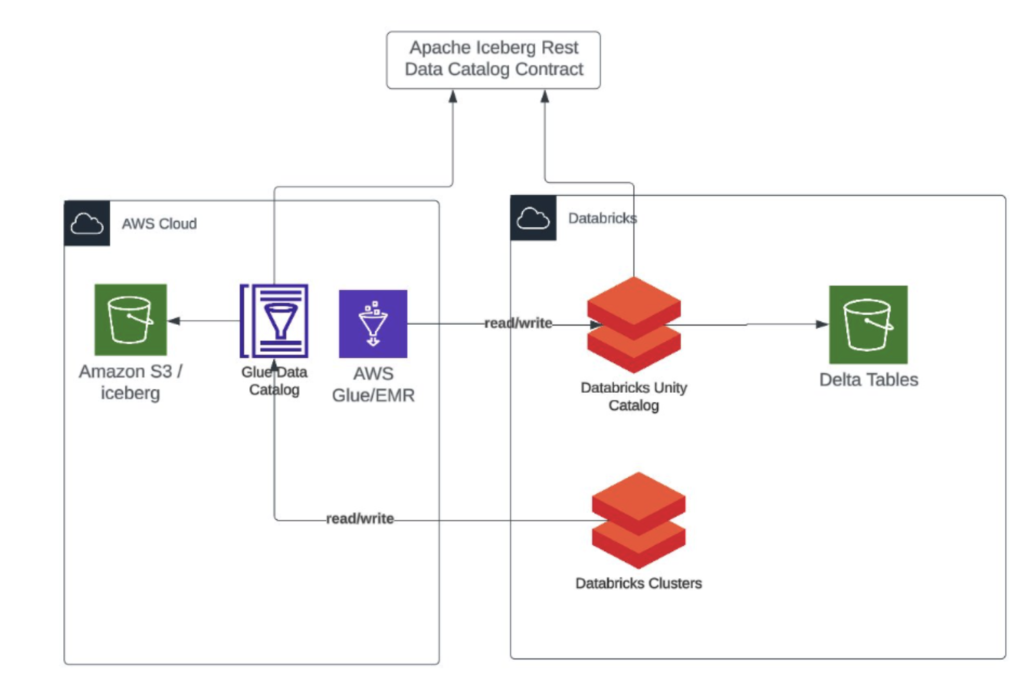
This marks a significant shift in how we approach cross-platform and multi-cloud data querying. What’s puzzling is why there now seems to be broad industry alignment on this paradigm—but regardless, it’s a promising step forward for data engineering. Looking ahead, I wonder if we’ll eventually see true separation of compute and storage across clouds.
This use case is particularly relevant for large enterprises operating across multiple clouds and platforms. For smaller companies, however, it may not be as critical.
What about Apache Hudi for cross query federation? It remains a significant platform, but I’m pessimistic about Apache XTable catching on. However, if your use case involves federated querying across a broader ecosystem, consider this design pattern.
Keep in mind that cross-platform read and write capabilities are being rolled out gradually. Be sure to conduct extensive proof-of-concept (POC) testing to ensure everything functions as expected.
Guidelines through data engineering technology selection
History is often a useful guide, and some are saying the Iceberg format will replace parquet files. As an example, a nifty feature is hidden partitioning, where you can change your partition and not have to rewrite your physical files in storage. The tradeoff is that Iceberg is not the simplest of platforms, you still do need to manage metadata and snapshots.
Here are some tips to guide your projects through a technology selection.
Does you actually have big data?
Big data is a hard term to define but typically think about organizations which have terabytes or petabytes of data. If they do qualify for this, you most likely will choose one of the 3 OTFs.
What ecosystem are you working in?
A fair amount of consideration should be given to the ecosystem and technologies they are working in.
- What programming languages does your staff know?
- Do they have any experience with any previous OTFs
What are the dimensions you need to balance?
There is a weird blending of datalakes and data warehouses now where the lines aren’t clear because features exist in both. We aren’t going to get clear lines soon, so consider these items.
- Cost At Scale
- After factoring in your projects expected growth patterns, which solution will scale the best at cost? For example, holding petabytes of data is probably better in a datalake than a data warehouse.
- Managed or Unmanaged AWS Service?
- It’s important to recognize that managed services, such as AWS Glue or MWAA, are not a one-size-fits-all solution. While they simplify operations, they also abstract away fine-tuning capabilities. Managed services often come at a higher cost but reduce operational complexity. When evaluating them, consider both infrastructure costs and the time and effort required for ongoing management.
- If your team has the engineering resources and expertise to manage an unmanaged platform, it may be a cost-effective choice at scale. If your project consists of a small team with limited bandwidth, opting for a managed AWS service can help streamline operations and reduce overhead.
Some members of the Hudi team argue that Open Table Formats aren’t truly “open.” While they raise a valid point, vendor lock-in hasn’t been a major concern for most projects. After all, choosing AWS or Databricks also involves a level of vendor lock-in. As a result, the arguments around openness may resonate more with a niche audience rather than the broader industry.
https://thenewstack.io/your-data-stack-is-outdated-heres-how-to-future-proof-it
Build Write-Audit-Publish pattern with Apache Iceberg branching and AWS Glue Data Quality
This is a really intriguing pattern for data quality. It enables you to test your data in a staging area, then commit it. This pattern is not necessarily new, but Iceberg’s branching features offers an easier way of doing this
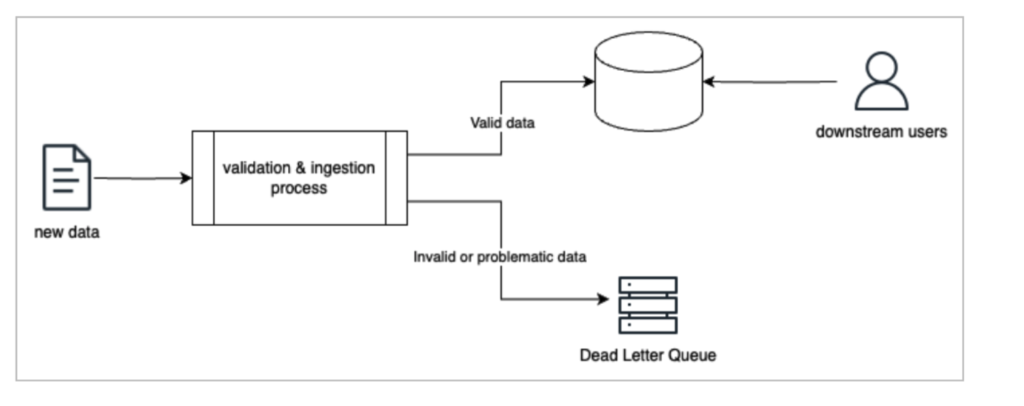
https://aws.amazon.com/blogs/big-data/build-write-audit-publish-pattern-with-apache-iceberg-branching-and-aws-glue-data-quality
https://lakefs.io/blog/data-engineering-patterns-write-audit-publish/
Implement historical record lookup and Slowly Changing Dimensions Type-2 using Apache Iceberg
Building a transaction datalake lake using Amazon Athena, Apache Iceberg and dbt
A case study how the UK Ministry of Justice saved quick a bit of money switching over to Iceberg using dbt
https://ministryofjustice.github.io/data-and-analytics-engineering/blog/posts/building-a-transaction-data-lake-using-amazon-athena-apache-iceberg-and-dbt/
Expand data access through Apache Iceberg using Delta Lake UniForm on AWS
Kind of a strange article, but there are still some customers using UniForm. With it, you can read Delta Lake tables as Apache Iceberg tables.
Amazon S3 Tables
One of the most significant announcements in the data space was Amazon S3 Tables. A common challenge with data lakes is that querying data in S3 can be slow. To mitigate this, techniques like compaction and partitioning are used to reduce the amount of data scanned.
Amazon S3 Tables address this issue by allowing files to be stored in S3 using the native Apache Iceberg format, enabling significantly faster query performance.
https://aws.amazon.com/blogs/aws/new-amazon-s3-tables-storage-optimized-for-analytics-workloads
However, there is a fair amount of criticism from competitors that there is a substantial mark-up in cost in this service, so be sure to read the S3 table pricing page
https://aws.amazon.com/s3/pricing
Redshift
Redshift History Mode – Redshift has released a new feature where if you have a zero ETL integration enabled, you can turn on Slowly Changing Dimensions (SCD) Type 2 out of the box.
Our historical pattern used to be
- DMS –> S3 (SCD Type 2) –> Spark job to reconcile SCD Type 1 or 2
Now we can do
- Zero ETL Redshift Replication –> Access SCD Type 2 tables
This is a relatively new feature so please do your comprehensive testing.
https://docs.aws.amazon.com/redshift/latest/mgmt/zero-etl-history-mode.html
Amazon Redshift now supports incremental refresh on Materialized Views (MVs) for data lake tables
https://aws.amazon.com/about-aws/whats-new/2024/10/amazon-redshift-incremental-refresh-mvs-data-lake-tables/
Apache Hudi Updates
Apache Hudi recently has reached a major milestone of having a 1.0.0 release in December, with several new features
A notable new feature allows users to create indexes on columns other than the Hudi record key, enabling faster lookups on non-record key columns within the table.
https://hudi.apache.org/blog/2024/12/16/announcing-hudi-1-0-0/#secondary-indexing-for-faster-lookups
https://www.onehouse.ai/blog/accelerating-lakehouse-table-performance-the-complete-guide
Glue
Glue 5.0
A newer version is out with faster runtimes, so be sure to use this version in your projects
https://aws.amazon.com/about-aws/whats-new/2024/12/aws-glue-5-0
New Glue Connectors
There are now 16 new connectors Adobe Analytics, Asana, Datadog, Facebook Page Insights, Freshdesk, Freshsales, Google Search Console, Linkedin, Mixpanel, Paypal Checkout, Quickbooks, SendGrid, SmartSheets, Twilio, WooCommerce and Zoom Meetings for Glue
https://aws.amazon.com/about-aws/whats-new/2024/12/aws-glue-16-native-connectors-applications
Glue Compaction with Mor Tables
Monitor and Manage Data Quality with Glue (Youtube Video)